Beyond the Box Score: How NBA Efficiency Ratings Reveal What Traditional Stats Hide
May 6, 2025 | by [email protected]
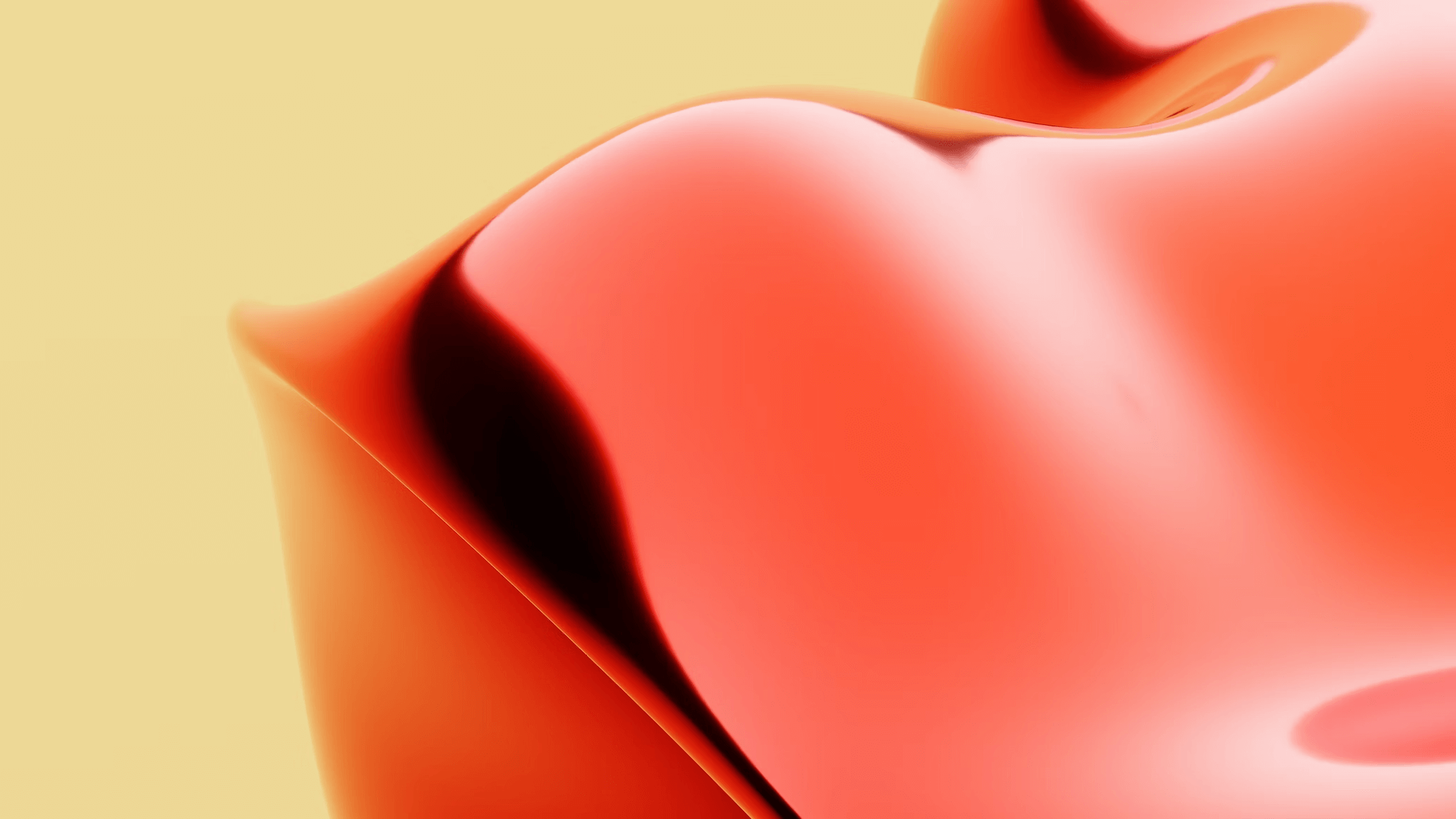
When you scan the morning box scores or glance at a player’s season averages, you’re only scratching the surface of basketball performance. Those traditional counting stats – points, rebounds, assists – that dominated basketball conversations for decades are increasingly recognized as incomplete measures of a player’s true impact. Enter the world of NBA efficiency ratings, sophisticated metrics designed to capture a player’s comprehensive value beyond what meets the casual eye.
In today’s analytics-driven NBA landscape, front offices, coaches, and savvy fans understand that traditional statistics can be actively misleading without proper context. A player scoring 25 points per game sounds impressive – until you learn they needed 30 shots to get there. As basketball analysis has evolved, efficiency-focused metrics now provide a deeper, more nuanced picture of performance that’s changing how we evaluate the game.
In this comprehensive guide, I’ll break down the most important efficiency metrics shaping NBA decision-making, explain how they work in practical terms, and show you why they’ve become essential tools for anyone who wants to truly understand basketball in 2025.
The Problem With Traditional Box Scores
When Counting Stats Deceive
Traditional basketball statistics have been the foundation of player evaluation for generations. Points, rebounds, assists, steals, and blocks – these counting stats are intuitive and easy to understand. They tell us what happened but not how efficiently it happened or within what context.
Consider two players:
- Player A: 22 points, 6-25 FG (24%), 8-8 FT
- Player B: 17 points, 7-12 FG (58%), 3-3 FT
If you just looked at points, Player A appears more productive. But Player B created nearly the same output while using far fewer possessions and shooting with remarkable efficiency. Traditional stats would mask this crucial difference – the basketball equivalent of comparing two cars solely by top speed while ignoring fuel economy.
The Empty Stats Phenomenon
The NBA landscape is littered with examples of “empty stats” players – those who compile impressive counting numbers on inefficient teams. These players often dominate possessions on losing teams, accumulating statistics that look impressive in isolation but don’t necessarily contribute to winning basketball.
The efficiency revolution in basketball analytics emerged partly as a response to this phenomenon. By measuring how effectively players turn possessions into points – basketball’s most valuable currency – advanced metrics help distinguish between volume production and valuable production.
As John Hollinger, creator of Player Efficiency Rating (PER), explains, these metrics allow us to “unify the disparate data on each player we try to track in our heads” to form a more complete picture of player impact.
Understanding Key Efficiency Metrics
Player Efficiency Rating (PER): The Pioneer Metric
PER stands as basketball’s first comprehensive efficiency metric to gain mainstream acceptance. Developed by John Hollinger (former ESPN analyst and later Memphis Grizzlies executive), PER attempts to boil down all of a player’s statistical contributions into a single number.
According to Wikipedia’s explanation of PER, this metric measures a player’s per-minute productivity while adjusting for pace. The formula incorporates positive accomplishments (field goals, free throws, 3-pointers, assists, rebounds, blocks, steals) and subtracts negative ones (missed shots, turnovers, personal fouls).
What makes PER particularly useful:
- It’s adjusted for minutes played, allowing comparisons between starters and bench players
- It accounts for team pace, so players on slow-paced teams aren’t penalized
- It’s normalized to a league average of 15.00, making cross-season comparisons possible
While PER isn’t perfect – it notably struggles to capture defensive impact beyond counting stats – it provides a quick reference point for overall production efficiency. A PER above 20 typically indicates an All-Star level performer, while values over 25 enter MVP territory.
True Shooting Percentage (TS%): The Complete Shooting Picture
While traditional field goal percentage treats all shots equally, True Shooting Percentage acknowledges the reality that some shots are worth more than others. This metric accounts for the added value of three-pointers and includes free throws in its calculation.
The formula: TS% = Points / (2 × (Field Goal Attempts + 0.44 × Free Throw Attempts))
The 0.44 multiplier for free throw attempts estimates the number of possessions used on free throws, since some trips to the line result from and-one situations or technical fouls.
TS% provides a significantly more accurate picture of shooting efficiency than field goal percentage alone. A player shooting 45% on mostly three-pointers is creating more value than someone shooting 48% exclusively on two-pointers – a distinction that traditional FG% would miss entirely.
Offensive and Defensive Efficiency Ratings
Individual Offensive Rating (ORtg) measures how many points a player produces per 100 possessions, while Defensive Rating (DRtg) estimates how many points they allow per 100 possessions. These metrics, pioneered by Dean Oliver (one of basketball analytics’ founding fathers), provide insight into a player’s impact on both ends of the floor.
Offensive Rating incorporates not just scoring but also assists, offensive rebounds, and other scoring-creating actions. Defensive Rating, though more estimate-based due to the collaborative nature of defense, attempts to quantify a player’s ability to prevent scoring using steals, blocks, defensive rebounds, and team defensive performance when they’re on the court.
The difference between these metrics – Net Rating – offers a quick glimpse into a player’s overall impact. A player with strong positive net ratings year after year is likely contributing significantly to winning, regardless of what their counting stats might suggest.
Beyond Individual Metrics: Team Efficiency
The Four Factors of Basketball Success
While individual efficiency metrics reveal player value, team success in basketball ultimately comes down to four key areas, according to analytics pioneer Dean Oliver:
- Shooting efficiency (measured by effective field goal percentage)
- Turnover rate (turnovers per possession)
- Rebounding percentage (percentage of available rebounds secured)
- Free throw rate (free throw attempts relative to field goal attempts)
Teams that excel in these four factors tend to win games consistently. Advanced analytics allow us to understand which players contribute positively to these crucial areas, often in ways traditional box scores miss entirely.
For instance, a player who creates spacing through excellent three-point shooting might not lead the team in points, but their impact on team offensive efficiency could be substantial by improving the team’s effective field goal percentage across the board.
Lineup Efficiency: The Ultimate Test
Perhaps the most telling efficiency measure is how the team performs with certain players or combinations on the court. Plus-minus statistics measure the scoring differential when a player is playing versus sitting.
Advanced versions like Adjusted Plus-Minus or Real Plus-Minus attempt to isolate a player’s impact by accounting for the quality of teammates and opponents. These metrics can reveal defensive specialists or offensive catalysts whose contributions might not show up in traditional statistics but who consistently help their teams outscore opponents.
The Evolution of Basketball Analytics
From Box Scores to Big Data
The journey from simple counting stats to sophisticated efficiency metrics represents a revolution in basketball analysis. This evolution mirrors similar developments in other sports, particularly baseball’s sabermetrics movement popularized by Bill James and others.
As explained in the Advanced Statistics in Basketball Wikipedia entry, basketball analytics (sometimes called APBRmetrics) applies rigorous statistical analysis to understand the game more objectively. Early pioneers like Dean Oliver, John Hollinger, and Justin Kubatko laid the groundwork for today’s analytics departments that exist in every NBA front office.
The modern NBA has embraced this analytical approach wholeheartedly. Teams now employ dedicated analytics staffs, tracking every imaginable aspect of the game:
- Player movement (distance, speed, acceleration)
- Shot quality based on defender distance
- Expected points from each possession type
- Defensive impact beyond traditional counting stats
This data-driven approach has fundamentally changed how teams evaluate talent, develop strategy, and make in-game decisions.
The Three-Point Revolution: Analytics in Action
No trend better illustrates the impact of efficiency metrics than the dramatic increase in three-point shooting across the NBA. Analytics clearly demonstrated that three-pointers, even at a lower conversion percentage, can be more efficient than most two-point attempts.
The math is simple: a 35% three-point shooter produces 1.05 points per attempt (0.35 × 3), while it takes a 52.5% shooter on two-pointers to match that efficiency (0.525 × 2 = 1.05). This realization has transformed basketball at all levels, with teams like the Houston Rockets at one point taking strategy to the extreme by almost entirely eliminating mid-range shots in favor of threes, layups, and free throws.
This strategic shift stems directly from efficiency metrics identifying the most valuable shot types – a clear example of how these advanced statistics have reshaped the game itself.
Case Studies: When Advanced Metrics Tell a Different Story
The Efficiency Stars Traditional Stats Missed
Some players’ contributions have been dramatically undervalued by traditional statistics throughout NBA history. Consider these examples:
Shane Battier: Often called “No-Stats All-Star,” Battier’s traditional numbers were unimpressive, but teams consistently performed better with him on the floor. Advanced metrics captured his defensive positioning, optimal shot selection, and team-enhancing play that box scores missed.
Draymond Green: Early in his career, Green’s counting stats rarely impressed, but his efficiency metrics – particularly defensive rating and adjusted plus-minus – identified him as a star-level contributor to championship teams well before casual observers recognized his value.
These players represent a category of contributors whose impact on winning far exceeds what traditional statistics suggest – something only revealed through efficiency-focused analysis.
Volume Scorers: When Traditional Stats Overvalue
Conversely, certain players throughout NBA history have compiled impressive scoring totals while advanced metrics painted a less favorable picture:
Allen Iverson: While undeniably talented and exciting, efficiency metrics suggested Iverson’s high-volume, relatively inefficient scoring approach (career 51.8% true shooting percentage, below league average) may have been less valuable than his point totals indicated.
Russell Westbrook: Despite triple-double seasons that looked historic in the box score, Westbrook’s efficiency metrics often told a different story. His below-average true shooting percentage and high turnover rate raised questions about how his style translated to team success.
These examples illustrate why teams now look beyond raw counting stats when evaluating player performance and making personnel decisions.
The Limitations of Efficiency Metrics
What the Numbers Can’t Capture
Despite their value, efficiency metrics aren’t perfect. Several important aspects of basketball remain difficult to quantify:
- Defensive impact beyond counting stats: While metrics attempt to capture defensive value, the interconnected nature of team defense makes isolation difficult.
- Gravity and spacing: A player’s mere presence affecting the defense (like Stephen Curry drawing defenders 30 feet from the basket) isn’t fully captured.
- Leadership and chemistry effects: How players influence team culture and cohesion remains largely outside statistical analysis.
- Context-specific value: Some players excel in specific roles or systems that efficiency metrics might not properly contextualize.
As with any analytical tool, efficiency ratings should complement rather than replace skilled observation and basketball intelligence. The most effective player evaluation combines quantitative analysis with qualitative understanding.
The Integration Challenge: Analytics and Traditional Evaluation
The basketball world has sometimes struggled to integrate advanced metrics with traditional evaluation methods. Analytics-focused approaches have occasionally been portrayed as being at odds with “old-school” basketball wisdom.
In reality, the most successful organizations blend both approaches. The analytics revolution hasn’t eliminated the need for scouting, film study, and basketball intuition – it’s enhanced these traditional methods with more precise, objective measurements.
As Watts Basketball explains, efficiency metrics provide a framework for judging a player’s impact beyond just their raw stats, but they work best when combined with contextual understanding of roles, systems, and team dynamics.
Efficiency Metrics: By the Numbers
Metric | What It Measures | League Average (Typical) | All-Star Level | MVP Consideration |
---|---|---|---|---|
PER | Overall per-minute productivity | 15.0 | 20+ | 25+ |
True Shooting % | Shooting efficiency including 3PT and FT | ~56% | 60%+ | 63%+ |
Offensive Rating | Points produced per 100 possessions | ~110 | 115+ | 120+ |
Defensive Rating | Points allowed per 100 possessions | ~110 | 105 or lower | 103 or lower |
Net Rating | Overall impact (ORtg – DRtg) | 0 | +5 or higher | +7 or higher |
My Thoughts: The Future of Basketball Evaluation
Having followed the evolution of basketball analytics for years, I’m convinced we’re still in the early stages of understanding how to properly evaluate player performance. The metrics discussed here represent significant improvements over traditional box scores, but the field continues to develop rapidly.
The next frontier likely involves better integration of tracking data (player movement, spacing effects) with traditional and current advanced statistics. We’re also seeing promising developments in measuring previously elusive defensive impacts through new camera and AI technologies.
What’s particularly exciting is how these advances are trickling down to all levels of basketball. Concepts like true shooting percentage and offensive efficiency that were once exclusive to NBA front offices are now commonplace in college programs and even high school teams.
For fans, understanding these metrics enhances appreciation of the game. A casual observer might miss the valuable contributions of a low-usage, high-efficiency role player or overvalue a high-scoring, inefficient volume shooter. Efficiency metrics provide the tools to recognize the difference.
Conclusion: The Full Picture of Basketball Value
Traditional basketball statistics served us well for decades, but they’ve always told an incomplete story. Efficiency metrics don’t replace the box score – they enhance it, providing crucial context about how effectively players convert their opportunities into team success.
As analytics continue reshaping basketball, the division between “eye test” and “analytics” evaluations is increasingly outdated. The most sophisticated basketball minds now recognize that efficiency metrics and traditional evaluation methods work best in tandem, each addressing the other’s blind spots.
Whether you’re a casual fan looking to better understand player value, a fantasy basketball manager seeking an edge, or a coach wanting to apply these concepts at any level, efficiency metrics provide a clearer, more complete picture of basketball performance than traditional statistics alone ever could.
FAQs: Understanding NBA Efficiency Metrics
1. Why is PER set to a league average of exactly 15.0 every season?
Setting PER to a consistent league average of 15.0 creates a stable reference point that allows for meaningful historical comparisons across different eras. This normalization accounts for changes in pace, rules, and playing styles over time, making it possible to compare a player from the 1990s to one playing today. It also creates an intuitive scale where above 15 means above-average productivity, and below 15 indicates below-average contribution.
2. Can efficiency metrics identify undervalued players for fantasy basketball or team construction?
Absolutely. Efficiency metrics often identify valuable contributors before their traditional statistics catch up. Players with high true shooting percentages, strong net ratings, and positive defensive metrics might be providing significant real-world value despite modest counting stats. In fantasy basketball, focusing on efficiency can help identify players who maximize categorical contributions (like FG%) while minimizing negatives (like turnovers), creating advantages that box-score hunters might miss.
3. How do teams use efficiency metrics in contract negotiations?
NBA front offices extensively use efficiency metrics to assess a player’s true value beyond their raw statistics. Teams may present evidence that a player’s efficiency metrics predict continued improvement or suggest decline, directly impacting contract offers. Players and agents likewise use favorable efficiency data to demonstrate value that traditional stats might understate. These metrics help both sides move beyond simple counting stats to more meaningful discussions of a player’s impact on winning basketball.
4. Do efficiency metrics favor certain player types or playing styles?
Some efficiency metrics do show patterns of favoritism. PER, for example, tends to rate high-usage scorers and rebounders more favorably than defensive specialists or low-usage role players. True Shooting Percentage naturally favors three-point specialists and players who draw frequent fouls. The most comprehensive player evaluation looks at multiple efficiency metrics together rather than relying on any single measure, which helps offset the biases inherent in individual statistics.
5. How can coaches use efficiency metrics to improve team performance?
Coaches increasingly use efficiency metrics to identify the most productive lineups, optimize shot selection, and develop game plans. By analyzing which five-player combinations produce the best net ratings, coaches can make data-informed rotation decisions. Efficiency metrics also help identify specific play types where a team excels or struggles, informing practice focus and strategic adjustments. At the individual level, these metrics help coaches provide targeted feedback for player development focused on improving efficiency rather than just raw production.
Citations:
- https://en.wikipedia.org/wiki/Player_efficiency_rating
- https://en.wikipedia.org/wiki/Advanced_statistics_in_basketball
- https://infographicsite.com/infographic/nba-player-performance-metrics-over-30-years/
- https://www.espn.com/nba/columns/story?columnist=hollinger_john&id=2850240
- https://wattsbasketball.com/blog/how-to-calculate-player-efficiency-rating
- https://www.nbastuffer.com/nba-player-efficiency-ratings-insights/
- https://www.nbastuffer.com/analytics101/nba-efficiency/
- https://www.reddit.com/r/nba/comments/3c75zk/why_do_people_seem_skeptical_of_advanced_stats/
- https://dataspace.princeton.edu/handle/88435/dsp01kk91fp74q
- https://www.reddit.com/r/nba/comments/z864c1/what_makes_an_nba_player_efficient/
- https://www.basketball-reference.com/about/ratings.html
- https://nbn23.com/improve-efficiency-basketball-statistics/
- https://www.basketballscience.net/explanations/context-is-key-stats-per-game-is-misleading
- https://jr.nba.com/basictraditional-stats-vs-advanced-stats/
- https://www.linkedin.com/pulse/nba-performance-metrics-learnings-pod-andrew-smith-khlfc
- https://www.youtube.com/watch?v=JsFRt_MNpDE
- https://www.basketball-reference.com/about/per.html
- https://en.wikipedia.org/wiki/Efficiency_(basketball)
- https://www.reddit.com/r/nbadiscussion/comments/cmrr8x/basketball_stat_player_efficiency_rating_per/
- http://20secondtimeout.blogspot.com/2013/11/charley-rosen-explains-why-statistics.html
Answer from Perplexity: pplx.ai/share
RELATED POSTS
View all