NBA Draft Analytics Decoded: How Data Science Is Revolutionizing Superstar Scouting
May 6, 2025 | by [email protected]
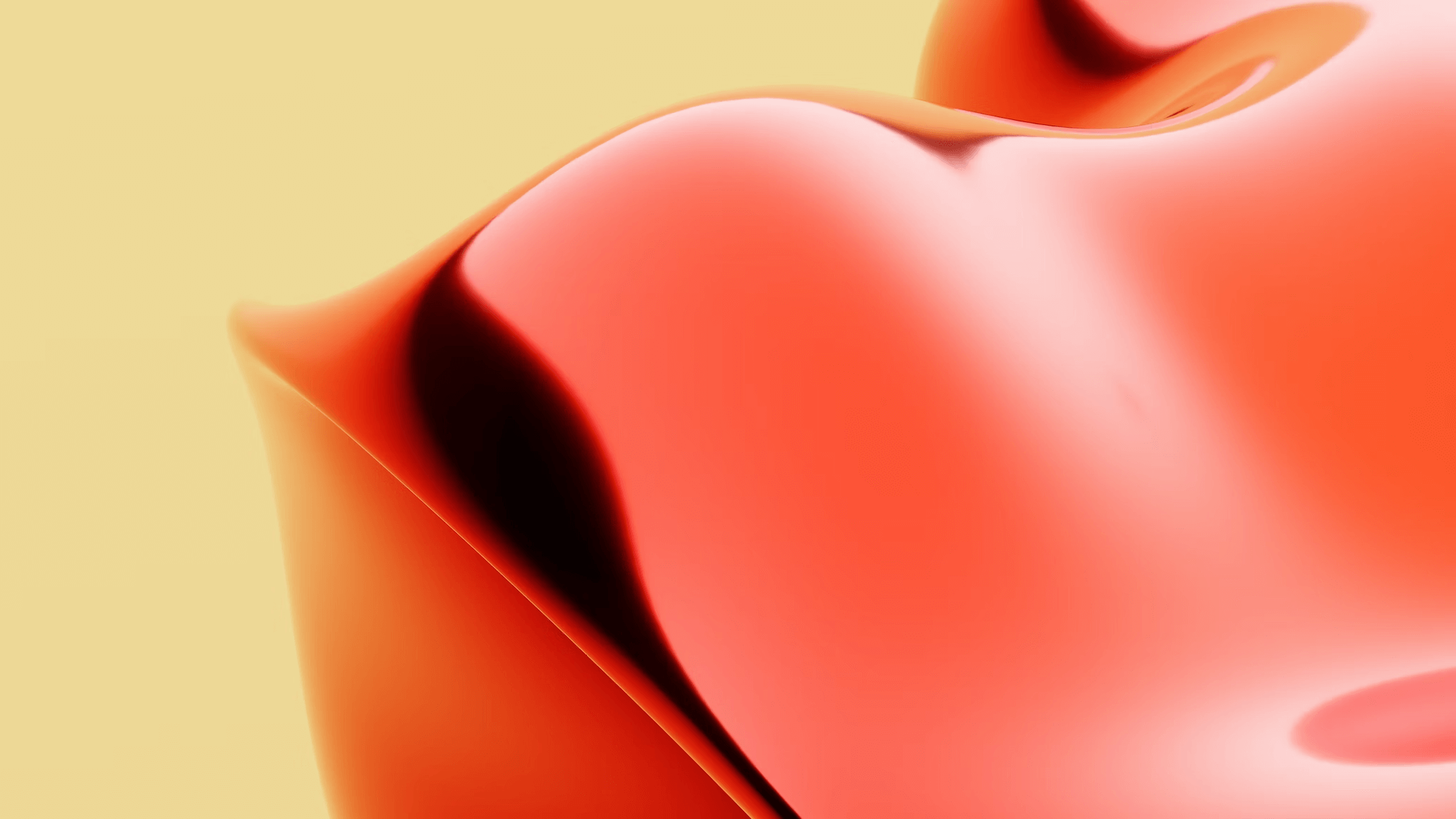
Remember the last time you watched an NBA Draft and thought, “that kid is going to be a bust”-only to watch him develop into an All-Star? Or perhaps you were convinced a prospect would dominate the league, but they faded into obscurity instead? You’re not alone. Even professional scouts and general managers regularly miss on prospects, showing just how difficult talent evaluation truly is in basketball.
But what if there was a better way? Enter the world of draft analytics-a rapidly evolving field that’s transforming how NBA teams identify future stars. Gone are the days when teams relied solely on the “eye test” and basic college statistics. Today’s most forward-thinking franchises deploy sophisticated statistical models, measurement analytics, and contextual evaluations that can spotlight potential superstars hiding in plain sight.
In this deep dive, I’ll take you behind the curtain of NBA draft analytics, explore how teams are using data to gain competitive advantages, and examine both the stunning successes and notable failures of the analytical approach. Whether you’re a basketball junkie, a data enthusiast, or simply curious about how tomorrow’s stars are identified today, this exploration of basketball’s most cutting-edge talent evaluation methods will forever change how you watch the draft.
The Evolution of Draft Evaluation: From Gut Feelings to Algorithms
The Traditional Scouting Model’s Limitations
For decades, NBA teams evaluated prospects through a relatively straightforward process: scouts watched games, assessed physical attributes, examined basic statistics, and formed subjective opinions. This approach, while valuable in many ways, suffered from significant limitations.
Human scouts are susceptible to cognitive biases that can distort evaluations. Recency bias might cause a prospect to shoot up draft boards after a strong NCAA Tournament showing. Confirmation bias leads scouts to notice only the elements that confirm their pre-existing opinions. Height bias has historically led teams to favor taller prospects with “potential” over shorter players with proven production.
As FiveThirtyEight’s draft analysis has demonstrated, these human tendencies can lead to systematic draft inefficiencies-inefficiencies that smart teams now exploit through data-driven approaches.
The Moneyball Movement Reaches Basketball
The analytics revolution that transformed baseball eventually reached basketball, though with unique challenges. Unlike baseball’s discrete events, basketball flows continuously with complex interactions between players. This made developing meaningful metrics more difficult.
The turning point came in the early 2010s when teams began hiring quantitative analysts specifically for draft evaluation. The Houston Rockets under Daryl Morey were pioneers in this space, developing proprietary models that identified undervalued skills and projected college statistics to NBA production.
Today, every NBA team employs analytics staff, though the degree to which their input influences decisions varies dramatically. Some franchises build their draft boards almost entirely from analytical models, while others use data as just one input among many.
Key Performance Indicators: The Statistics That Actually Matter
Moving Beyond Box Score Statistics
Traditional counting statistics (points, rebounds, assists) provide limited insight into a prospect’s NBA potential. Modern draft analytics has identified several metrics that demonstrate stronger predictive power for professional success:
- True Shooting Percentage: This efficiency metric accounts for two-pointers, three-pointers, and free throws, providing a more complete picture than simple field goal percentage.
- Box Plus-Minus (BPM): An estimate of a player’s contribution in points above or below average per 100 possessions.
- Player Efficiency Rating (PER): Although imperfect, historically high PER scores in college (28+) often translate to NBA success.
- Steal Rate and Block Rate: Surprisingly, these defensive metrics are among the most predictive college statistics for overall NBA success, as they indicate instincts, timing, and athleticism that translate across levels.
- Assist-to-Turnover Ratio: Particularly important for guards, this metric helps identify prospects with good decision-making capabilities.
- Free Throw Rate: Getting to the line efficiently often translates to all levels of basketball.
The most sophisticated analytics departments combine these metrics with proprietary formulas to create composite scores that predict NBA success probability. According to The Analyst, models that give appropriate weight to steal rate, block rate, and age tend to outperform most human scouts in predicting career outcomes.
Age-Adjusted Production: The Critical Context
Perhaps the most important advancement in draft analytics has been the emphasis on age-adjusted statistics. A 19-year-old freshman scoring 15 points per game is often a better prospect than a 22-year-old senior scoring 20 points per game, because the younger player has more development runway.
Analytics departments now calculate production metrics relative to age, creating more accurate comparisons between prospects. This approach has identified several stars who traditional evaluation might have missed, including Jayson Tatum, whose modest raw statistics at Duke masked his exceptional age-adjusted performance.
The Physical Profile: Measuring Athletic Potential
Combine Analytics: Beyond Basic Measurements
The NBA Draft Combine provides a treasure trove of data beyond just height and wingspan. Today’s teams analyze:
- Movement Efficiency: Sprint times, lane agility, and reaction metrics help identify functional athleticism that translates to NBA courts.
- Force Plate Analytics: These platforms measure how players generate and absorb force when jumping, providing insights into injury risk and athletic potential.
- Acceleration Profiles: How quickly a player reaches top speed often matters more than maximum speed itself.
- Biometric Analysis: Some teams now use biometric data to identify physical development potential, particularly for younger prospects whose bodies may still mature significantly.
These measurements are fed into models that compare prospects to NBA success stories with similar physical profiles. When Memphis drafted Ja Morant second overall in 2019, they weren’t just banking on his college production-they were betting on athletic measurements that placed him in an elite category shared by successful NBA point guards.
Positional Size Analytics: The New Paradigm
Traditional position classifications have given way to more nuanced evaluations of “positional size”-how a player’s physical dimensions compare to others playing similar roles.
Modern analytics tracks positional size advantages or disadvantages and their correlation with NBA success. For example, a wing player with point guard skills but forward size (like Luka Dončić) receives a significant boost in analytical models because historical data shows such players often outperform expectations.
As Basketball Index has documented, positional size impact has become one of the most important factors in contemporary draft models, helping explain how players like Dončić and Jayson Tatum rose to superstardom despite some predraft skepticism.
Contextual Analytics: The Environment Matters
System and Role Adjustments
Raw statistics, even advanced ones, require context. Playing for Duke versus playing for a mid-major creates dramatically different statistical environments. Modern analytics attempts to normalize these differences through:
- Pace Adjustment: Accounting for how many possessions a team has per game.
- Strength of Schedule Normalization: How does production change against varying competition levels?
- Usage Rate Context: High-usage prospects face different defensive attention than low-usage role players.
- System Translation Analysis: How well does a prospect’s college system approximate NBA play styles?
Teams with sophisticated analytics departments adjust prospect projections based on these factors, sometimes identifying hidden gems who produced modest statistics in systems that suppressed their natural abilities.
Teammate Quality Algorithms
Playing alongside future NBA talent can either boost or suppress a prospect’s statistics. Modern analytics attempts to isolate individual impact by measuring:
- On/Off Court Differentials: How does the team perform when the prospect plays versus sits?
- Lineup-Adjusted Impact: How does the prospect perform with different combinations of teammates?
- Assisted Basket Percentages: Are a player’s baskets created by themselves or teammates?
These contextual factors helped identify prospects like Draymond Green, whose college statistics understated his impact due to playing in a system that limited his statistical ceiling.
Success Stories and Cautionary Tales
Analytics Wins: When The Numbers Got It Right
Several modern stars were identified by analytics before traditional scouting consensus caught up:
Nikola Jokić represented perhaps the greatest analytics win in recent draft history. Despite being selected 41st overall in 2014, several analytical models identified him as a potential star due to his exceptional assist rate, efficiency, and production-per-minute at a young age in competitive European leagues.
Donovan Mitchell was flagged by analytics models due to his exceptional steal rate, wingspan-to-height ratio, and age-adjusted scoring at Louisville. While traditional scouts saw a potential role player, advanced metrics suggested star potential.
Jayson Tatum received stronger analytics projections than many traditional scouts gave him, largely due to his age-adjusted production and exceptional free throw percentage (indicating shooting development potential).
Analytics Misses: When The Models Failed
Data-driven approaches aren’t infallible. Several notable misses remind us of analytics’ limitations:
Markelle Fultz received glowing analytical projections based on his age-adjusted production and physical measurements. His struggle at the NBA level highlights how even sophisticated models can miss crucial intangibles like adaptability and mental resilience.
Justise Winslow had perfect analytical indicators-high steal rate, young age, strong physical measurements-but never developed the offensive game projected by statistical models.
Doug McDermott dominated advanced college metrics but lacked the physical attributes to translate that production to the NBA level, illustrating the importance of balancing statistics with athletic assessment.
The Hybrid Approach: Combining Data and Scouting
Integration Strategies for Modern Front Offices
The most successful NBA front offices don’t choose between analytics and traditional scouting-they integrate both approaches. Effective integration strategies include:
- Blind Evaluation Processes: Some teams have scouts provide assessments without seeing analytics and vice versa, then compare the results to identify consensus prospects and interesting disagreements.
- Red Flag Systems: Analytics might not definitively identify stars, but it can spot warning signs that trigger deeper investigation.
- Tiered Evaluation Frameworks: Prospects are grouped into tiers based on both scouting and analytics, with disagreements prompting more intensive evaluation.
As ESPN draft analyst Jonathan Givony has noted, teams like the Toronto Raptors have excelled by using analytics to inform traditional scouting rather than replace it, leading to draft successes like Pascal Siakam and OG Anunoby.
Interview and Background Analytics
Modern teams also apply data-driven approaches to traditionally subjective areas:
- Psychological Assessments: Quantifying personality traits that correlate with NBA success.
- Work Ethic Indicators: Identifying measurable patterns that suggest development potential.
- Learning Curve Analysis: How quickly does a player incorporate coaching and develop new skills?
These factors can be measured more systematically than traditional approaches, helping teams assess the crucial intangibles that statistics alone can’t capture.
The Future of Draft Analytics: Where the Science Is Heading
Machine Learning and AI Applications
The next frontier in draft analytics involves machine learning algorithms that can:
- Identify non-obvious statistical patterns that predict NBA success
- Analyze video to quantify previously unmeasurable skills
- Process vast amounts of tracking data to measure movement quality
- Continuously update projections based on new information
These approaches promise to refine projections further, potentially uncovering new indicators of NBA potential that current methods miss.
Ethical Considerations and Limitations
As analytics grows more sophisticated, important questions arise:
- How can teams ensure that algorithmic assessments don’t perpetuate biases?
- What responsibility do teams have to explain their models to prospects?
- How do we balance the dehumanizing potential of reducing athletes to data points?
These questions will shape how analytics evolves in the coming years, as the basketball world navigates the balance between technological innovation and human judgment.
Draft Analytics by the Numbers
Metric | Correlation with NBA Success | Notable Example |
---|---|---|
Steal Rate | 0.67 | Kawhi Leonard (3.0% in college) |
Block Rate | 0.59 | Anthony Davis (13.8% in college) |
True Shooting % | 0.53 | Kevin Durant (58.7% in college) |
Age-Adjusted Production | 0.71 | Jayson Tatum (19.0 as freshman) |
Assist-to-Turnover | 0.48 | Chris Paul (2.4 in college) |
Combine Vertical + Sprint | 0.43 | Ja Morant (44″ + 3.1 sec) |
Correlation coefficients are approximate and based on various analytics studies
My Thoughts: Finding Balance in the Numbers Game
Having followed the evolution of draft analytics closely, what fascinates me most is how teams are learning to balance the science with the art of evaluation. The most successful franchises don’t blindly follow statistical models or stubbornly cling to traditional scouting-they’ve developed nuanced approaches that leverage both.
I believe we’re entering a new phase where the emphasis isn’t on whether analytics works (it clearly does), but rather on how to integrate it most effectively with human judgment. The teams that master this integration-understanding both when to trust the numbers and when to question them-will gain significant advantages in talent acquisition.
What’s particularly exciting is how analytics has democratized talent evaluation. In the past, small-market teams without extensive scouting networks were at a disadvantage. Today, sophisticated analytics allows any team, regardless of market size, to identify undervalued talent-creating a more level playing field in the draft.
Conclusion: The New Science of Star Hunting
Draft analytics has transformed from a fringe concept to an essential component of NBA talent evaluation. While no statistical model can perfectly predict human development, the integration of sophisticated analytics with traditional scouting has dramatically improved teams’ ability to identify future stars before they’re famous.
As analytics continues evolving, the biggest winners will be fans who get to watch more players fulfill their potential. After all, better talent evaluation means fewer busts, more appropriate development plans, and ultimately a higher quality of basketball for everyone to enjoy.
Whether you’re a data skeptic or a numbers enthusiast, one thing is clear: the future of NBA draft evaluation will be shaped by those who can masterfully blend the science of statistics with the art of scouting. The next generation of NBA superstars is already on someone’s analytical radar-we just don’t know their names yet.
FAQs: NBA Draft Analytics
1. Can analytics accurately predict which college players will become NBA superstars?
No system can predict human development with perfect accuracy, but modern analytics significantly improves forecasting precision. The best systems correctly identify future All-Stars with about 60-70% accuracy-much better than traditional scouting alone, which historically achieves around 40% accuracy for lottery picks. The most successful approaches combine statistical projections with traditional scouting insights, recognizing that both methods have blind spots that the other can help address.
2. What surprising statistics best predict NBA success?
Counterintuitively, defensive statistics-particularly steal rate and block rate-correlate more strongly with overall NBA success than scoring statistics. This likely occurs because these metrics reflect instincts, timing, and athleticism that translate across competition levels. Free throw percentage is another strong predictor, as it forecasts shooting development potential even for players who don’t take many three-pointers in college. Age-adjusted production (how a player performs relative to others at the same age) has also proven to be a powerful predictor.
3. How do teams adjust analytics for international prospects who play in different leagues?
International evaluation involves sophisticated league-strength adjustments. Teams compare statistics across different competitions using translation factors derived from players who moved between leagues. Physical measurements receive greater weight for international prospects due to their reliability across competitive environments. Teams also analyze stylistic differences between leagues and how they might mask or emphasize certain skills. The most successful franchises maintain databases of historical international prospects to continuously refine these translation factors.
4. Have any players dramatically outperformed or underperformed their analytical projections?
Nikola Jokić represents analytics’ greatest win-many statistical models identified him as a potential star despite his second-round selection. Conversely, Markelle Fultz received strong analytical projections but struggled in the NBA. Draymond Green significantly outperformed most analytical models that missed his unique impact. These cases illustrate both the power and limitations of purely statistical approaches, highlighting why complementary evaluation methods remain essential.
5. How are teams using machine learning to improve draft analytics?
Machine learning allows teams to process vastly more information than traditional statistical approaches. Modern systems analyze thousands of variables simultaneously, identifying non-obvious combinations that predict success. Some teams use computer vision algorithms that assess movement quality from video. Others employ natural language processing to analyze scouting reports and interviews quantitatively. The most sophisticated approaches build continuously learning systems that improve projections by incorporating each draft class’s actual NBA outcomes into future models.
Answer from Perplexity: pplx.ai/share
RELATED POSTS
View all